Post Views: 317
For the last six years (starting in 2018), AI investors Nathan Benaich and Air Street Capital have consistently published the State of AI Report. This article summarizes key takeaways from the 2024 report published here, along with the author’s interpretation of model cards, industry reports, research reports, and more available publicly.
#1 – Frontier lab performance converges, but OpenAI maintains its edge following the launch of o1 (aka Strawberry)
- For much of the year, both benchmarks and community leaderboards pointed to a chasm between GPT-4 and ‘the best of the rest’. However, Claude 3.5 Sonnet, Gemini 1.5, and Grok 2 have all but eliminated this gap as model performance now begin to converge as per the announcement:
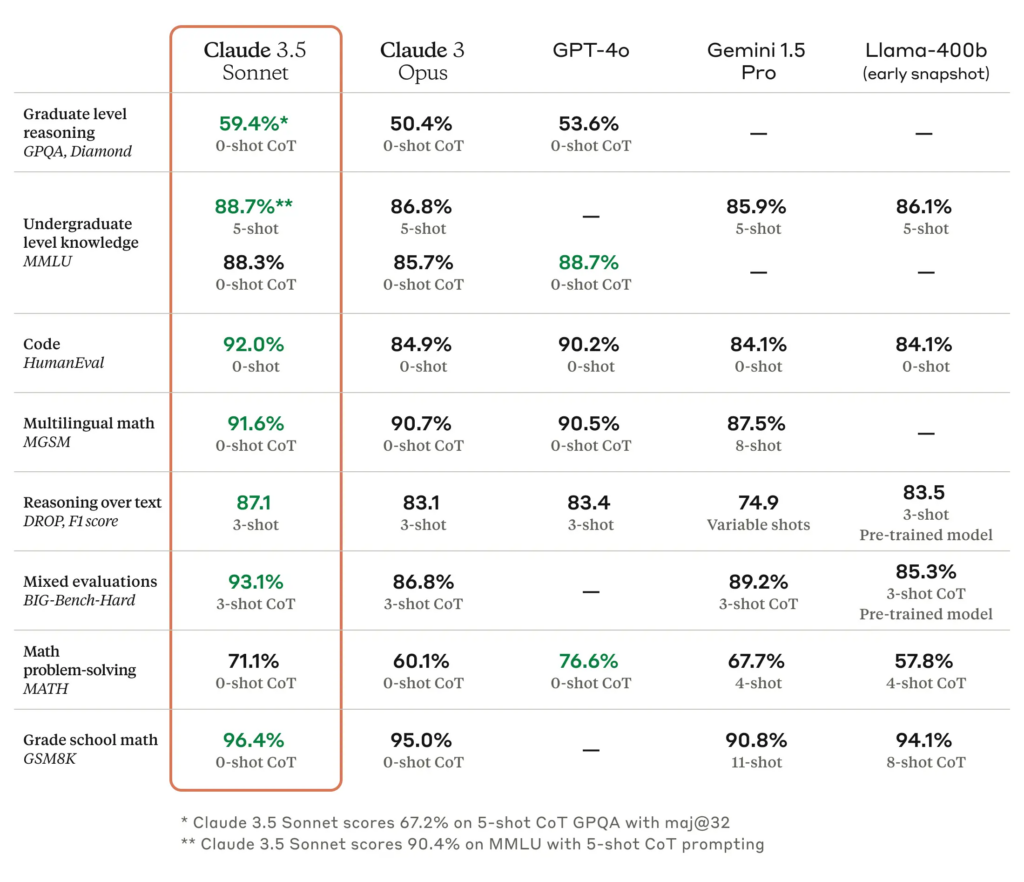
- By shifting compute from pre- and post-training to inference, OpenAI o1 reasons through complex prompts step-by-step in a chain-of-thought (COT) style.
- Researchers have increasingly been shining a light on dataset contamination, and we need to be careful about interpreting reports published by new models with strong benchmark performance. As per the Scale paper, Gemini and OpenAI GPT models show no sign of overfitting (Overfitting a model is a machine learning problem that occurs when a model is too closely aligned with its training data):
When benchmarking leading open- and closed-source models, we find substantial evidence that many models have been contaminated by benchmark data, with models showing performance drops of up to 13% accuracy. We notice that Mistral and Phi top the list of overfit models, with almost 10% drops on GSM1k compared to GSM8k, while models such as Gemini, GPT, and Claude show little to no signs of overfitting.
Source: Scale Report
1.1 – The top quality model, OpenAI’s o1, comes at a significant price and latency premiums
#2 – Llama 3 closes the gap between open and closed models, but the definition of openness plays a vital role
- Llama 3.1 405B, their largest to-date, is able to hold its own against GPT-4o and Claude 3.5 Sonnet across reasoning, math, multilingual, and long-context tasks.
- With open source commanding considerable community support and becoming a regulatory issue, some researchers have suggested that the term is often used misleadingly. It can be used to lump together vastly different openness practices across weights, datasets, licensing, and access methods. See a comparative view of in terms of various aspects of openness of 40 text generation models have been assessed as part of the research below:
#3 – NVIDIA remains the most powerful company in the world, enjoying a stint in the $3T club
- Driven by a bull run in public markets, AI companies reach $9T in value, while investment levels grow healthily in private companies.
- NVIDIA remains the most powerful company in the world, enjoying a stint in the $3T club, while regulators probe the concentrations of power within GenAI.
- A handful of challengers demonstrate signs of traction such as Cerebras, Groq
#4 – Rise of high-performing LLM and multimodal models that are small enough to run on smartphones
- Microsoft’s phi-3.5-mini is a 3.8B LM that competes with larger models like 7B and Llama 3.1 8B.
- Apple introduced MobileCLIP, a family of efficient image-text models optimized for fast inference on smartphones.
- Hugging Face also got in on the action with SmolLM, a family of small language models, available in 135M, 360M, and 1.7B formats.
On the other hand, AI researchers have been conducting experiments to find their replacements. Refer to the research published by Sakana.ai), which introduces, The AI Scientist, is an end-to-end framework designed to automate the generation of research ideas, implementation, and the production of research papers:
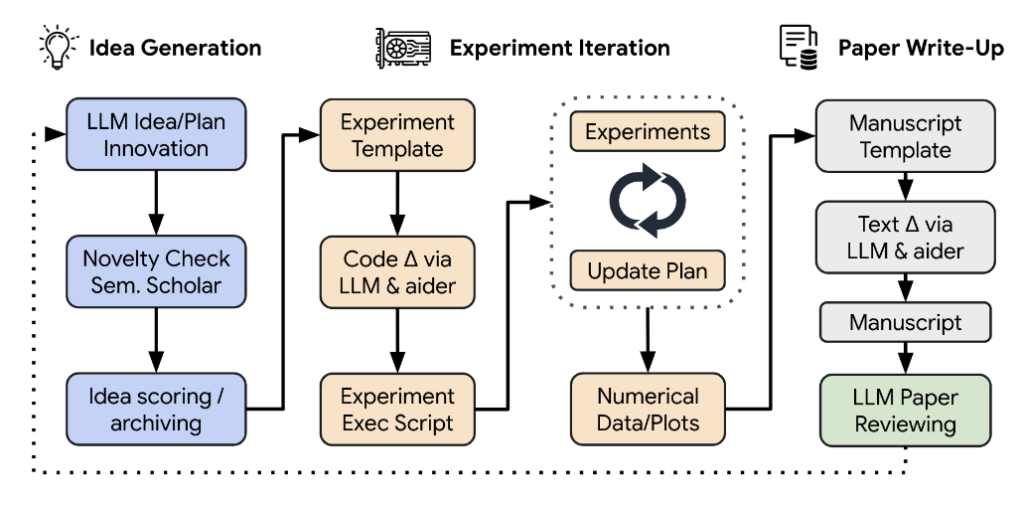
#5 – Government regulation and safety remains an open challenge at a global level
- While global governance efforts stall, national and regional AI regulation has continued to advance, with controversial legislation passing in the US and EU.
- Anticipated AI effects on elections, employment and other sensitive areas are yet to be realized at any scale.
- Every proposed jailbreaking ‘fix’ has failed, but researchers are increasingly concerned with more sophisticated, long-term attacks.
- Governments worldwide emulate the UK in building up state capacity around AI safety, launching institutes and studying critical national infrastructure for potential vulnerabilities.
Additional Insights:
- Hybrid models begin to gain traction (such as Falcon’s Mamba 7B, AI21’s Mamba-Transformer, Griffin, trained by Google DeepMind)
- Synthetic data starts gaining more widespread adoption.
- Web data is decanted openly at scale proving quality is key and prompted improvements in the quality of embedding models.
- Context proves a crucial driver of performance. For example, Anthropic solved this using ‘contextual embeddings’, where a prompt instructs the model to generate text explaining the context of each chunk in the document.
- Retrieval and embeddings hit the center stage.
- VLMs achieve SOTA performance out-of-the-box.
- Hugging Face pulls down barriers to entry.
- Evaluation for RAG remains unsolved – Researchers are now pioneering novel approaches, like Ragnarök.
- Enterprise automation set to get an AI-first upgrade considering the cost of RPA in the past.
- It’s possible to shrink models with minimal impact on performance (e.g. NVIDIA researchers took a more radical approach by pruning layers, neurons, attention heads, and embeddings, and then using knowledge distillation for efficient retraining.)
- Github reigns supreme, but an ecosystem of AI coding companies is growing (Anysphere, Cognition, Magic, Qudo)
Disclaimer:
All data and information provided on this blog are for informational purposes only. The author makes no representations as to the accuracy, completeness, correctness, suitability, or validity of any information on this blog and will not be liable for any errors, omissions, or delays in this information or any losses, injuries, or damages arising from its display or use. This is a personal view and the opinions expressed here represent my own and not those of my employer or any other organization. The interpretation of report is based on the author’s determination based on the assessment of the content – there might be differences of opinion, which can’t be attributed back to the author.